The datafication of music and its implications on mental well-being
Author(s): City Air NewsMental illness identification in India gets a boost with non-intrusive methods developed by music psychology researchers at IIIT Hyderabad. It was while streaming Coldplay in an endless loop that I first discovered...
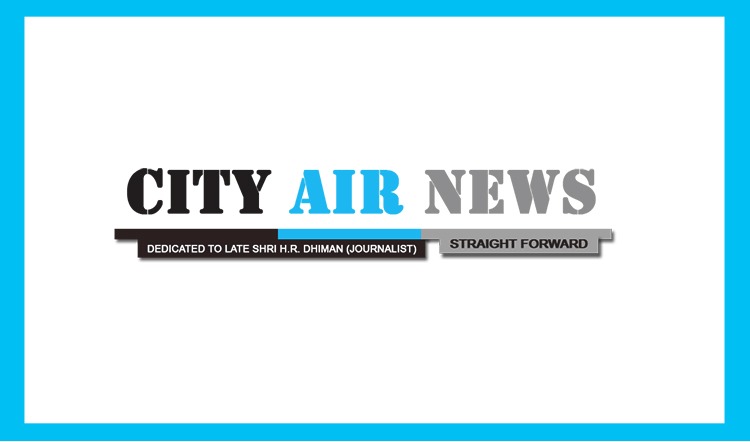
Mental illness identification in India gets a boost with non-intrusive methods developed by music psychology researchers at IIIT Hyderabad.
It was while streaming Coldplay in an endless loop that I first discovered Kodaline. A suggested playlist that popped up based on my listening history seemed to exhort me to consider Steve Garrigan’s soulful voice. Needless to say, it was pretty spot on! This is what data analytics in music does. Based on user behaviour, it is easy to tell what kind of people like to listen to what kind of music, at what kind of location, at what time, and so on. But personalizing the entire music listening experience is just one part of the story. Big data hugely benefits the music industry by analysing listening patterns, preferences and musical genres to predict which song is likely to become a runaway hit. And now, going a step further from analyzing metrics for business purposes, is newer research that proposes mining such music data and finding insights into people’s internal states.
Music and Big Data: A New Frontier
In a paper by David Greenberg and Peter Rentfrow, Music and Big Data: A New Frontier, the authors say, “There are now streaming services that store data from millions of people on their day-to-day musical listening habits; song-level data that tags sonic and emotion attributes for millions of songs; wearable devices (e.g. watches and earbuds) that capture physiological metrics including heartrate and galvanic skin response; mobile technologies that track a person's moment-to-moment activity, location, mood, and sociability; and survey instruments and digital footprints that capture personality and other biopsychosocial metrics in just under a minute.” They propose that a combination of all these technologies can usher in a new era for Music Psychology. Dr. Vinoo Alluri who leads research on Music Cognition at the Cognitive Science lab at IIITH agrees. Citing existing research which found that personality even predicts words in favourite songs (Qui, Chen, Ramsay et al), Dr. Alluri says, “There is sufficient evidence that supports the concept that music (rather musical choice) is indeed a mirror of the self and that it is an emotional, social, and cognitive expression of who we are. Also, our current states dictate the choice of music we would like to listen to (for ex: if we feel grumpy versus happy, we might pick different kinds of music to listen to). But now emerging research is demonstrating how music plays a role as a companion, a social surrogate of sorts, and serves a purpose other than just to relax as we think of music in India.”
Healthy-Unhealthy Music Scale
In 2015, to probe what music listening strategies reveal about our current mental state, Saarikallio et al conducted a study on Australian adolescents. They interviewed adolescents suffering from depression in a clinical setting and came up with a 13-point questionnaire that is widely known as HUMS or the Healthy Unhealthy Music Scale. Some of the examples of ‘Healthy’ items on the scale are ‘Music helps me relax’, ‘I feel happier after playing or listening to music’ and so on. Some of the ‘Unhealthy’ items are ‘I hide in my music because nobody understands me, and it blocks people out’, ‘I like to listen to songs over and over, even though it makes me worse’, and so on. Researchers found that those who scored high on HUMS unhealthy items also scored high on the standard Kessler Psychological Distress Scale (K10). And in turn, they scored low on standard mental wellbeing diagnostic measures. “On the distress scale, if you are above a certain threshold, you could be considered high risk; in the sense that you either already have some mental issues, or you are at a high risk of developing them,” explains Dr. Alluri. The researchers concluded that HUMS is not a direct measure of mental ill-being but an instrument to detect the risk of potential mental health problems via a non-intrusive way. And a high HUMS unhealthy score could be followed up with a screening measure for depression and suicidal ideation to conclusively find out.
An Indian Setting: Enter ML
To check the validity of HUMS in the Indian context, Dr. Alluri and her students first tested it out on young adults whose average age was 24. “There were no differences in the results. We found very similar patterns,” says Dr. Alluri. Then they tried it in an IT setting where 151 respondents working for an IT company were sampled. Again highly similar results were exhibited. “It shows that no matter the age, if people are going through some distress, their musical engagement strategies are similar,” explains Dr. Alluri. While the original Australian study sought to find a correlation between HUMS and the Kessler scale, Dr. Alluri and her students, Rajat Agarwal and Ravinder Singh, applied machine learning approaches to make accurate predictions of mental well-being from music associations. It was found that regardless of the dataset used, a universal pattern emerged allowing the researchers to predict mental well-being with 81% accuracy.
Significance
Explaining the significance of their research in the Indian context, Dr. Alluri says, “In India, people do not want to talk about mental illness due to the stigma associated with it. Some of the standard diagnostic tools to assess mental illness may have intrusive questions like, “In the past 2 weeks, did you have thoughts that you would be better off dead, or of hurting yourself in some way?’ and so on. It is not easy for people to acknowledge that. So we’re using this as an indirect way to identify risk of depression.” The findings were published in a paper titled ‘Mining Mental States Using Music Associations” which was accepted recently for the Speech, Music, and Mind with Audio Satellite Workshop held at InterSpeech 2019, in September in Austria. It is labelled as the world‘s largest and most comprehensive conference on the science and technology of spoken language processing.
Current Study With Music Streaming
On the lines of Greenberg and Rentfrow’s paper on big data and music, researchers at IIITH too are trying to find answers to the bigger question of whether it is possible to unobtrusively monitor music listening preferences to identify and pre-empt red flags in mental health. Towards this end, they have begun analysing time stamps of 600 willing participants who are last.FM (a music streaming platform) users. In addition they have also obtained their HUMS and personality scores. “Last.Fm API allows us to mine music listening history which allow us to obtain information ranging from semantics (tags, lyrics) to information about the music (genre, artist, etc.) to the acoustic content of the song, to users’ listening patterns (if they are listening to the same set of artists on a regular basis or sporadically). Now we are trying to understand what sort of patterns (either textual, acoustic, or habitual) exist in a more realistic music listening setting which may be indicative of poor mental health in addition to uncovering the potential modulatory role of personality” says Dr. Alluri. While conclusive results are awaited, it is a big step towards overcoming current taboos about mental health that exist in Indian society.